Essentially, data science entails the incorporation of machine learning methods in conjunction with the use of analytics to extract value from data . As a consequence of the increased volume of data, improved machine learning algorithms, and greater computer resources, there is presently a tsunami of expansion rolling across the area of data science.
Because of the essential characteristics and impacts that data science has had and will proceed to exert on our lives, this entire article will explain the importance of data science, the trends going to lead to the coming years of data science, and how businesses & individuals can sufficiently anticipate the future.
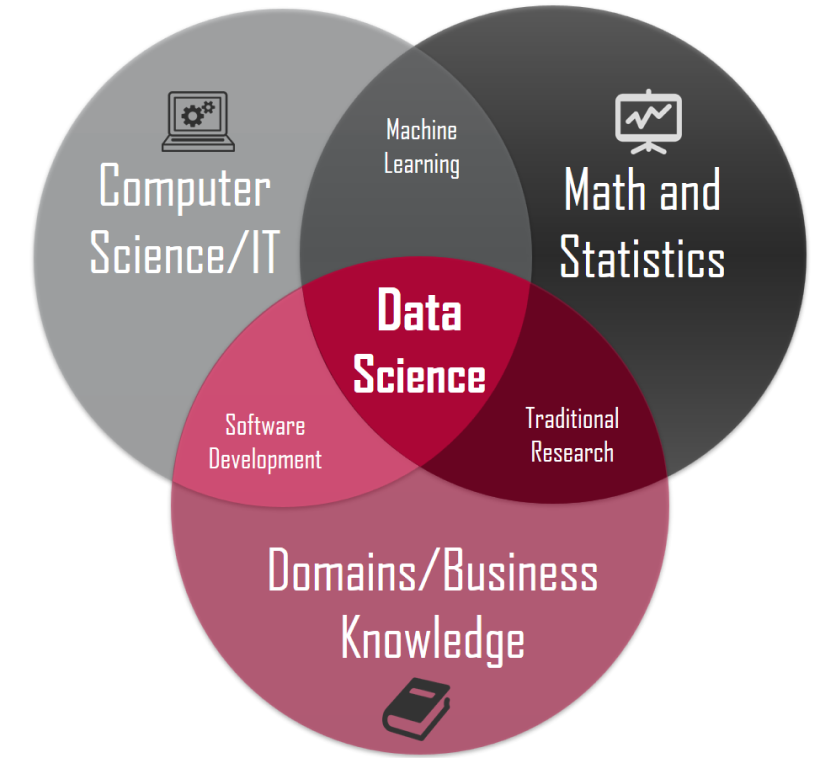
12 Industry Trends That Will Shape Data Science's Future
Rapidly Expanding Internet of Things Networks (IoT)
In the coming years, the Internet of Things (IoT) will be a fundamental engine of digital transformation. According to IDC, global IoT spending will grow at a CAGR of 13.6% from 2017 to 2022, surpassing $1 trillion in 2022.
According to Ericsson, the number of mobile IoT connections will reach 3.5 billion by 2023, growing at a CAGR of 30%. The expanding IoT trend is attracting more and more enterprises to invest, with smart gadgets including Apple Siri, Microsoft Cortana, Amazon Alexa, and Google Home emerging in households to streamline and automate certain chores. Every implementation plan should consider what will be done with potential data acquired when firms implement IoT technologies.
IoT will shape the future of Data Science by propelling new tools and tactics capable of processing massive amounts of data.
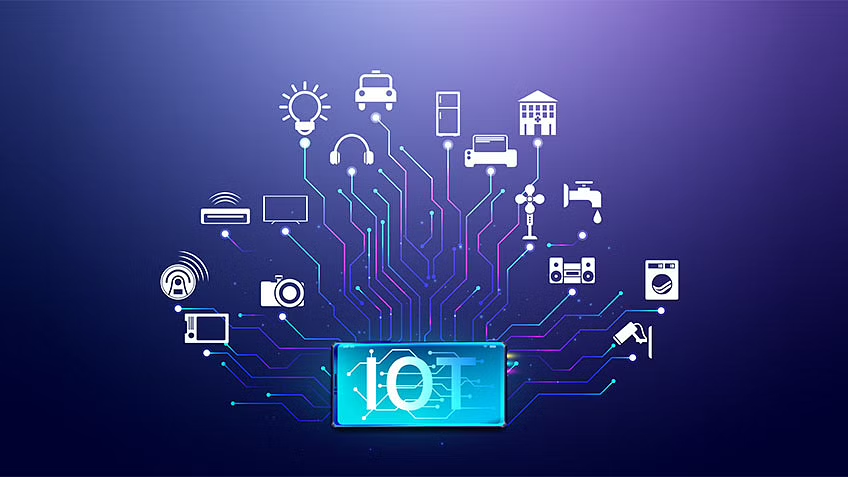
Creating Digital Assets from 'Shadow/Dark Data'
A considerable amount of the obtained data has not yet been converted to digital format, implying that it has not yet been examined. IBM estimates that 80% of all data comes from largely untapped, unstructured text/programmed material. This unstructured data, dubbed 'Dark Data,' is expected to be turned into digital assets since it contains previously unknown and essential insights and constitutes a missed opportunity. To capitalize on the promise of shadow data, a growing number of data researchers will focus on 'dark analytics,' for which enhanced tools & new professional skills will be required.
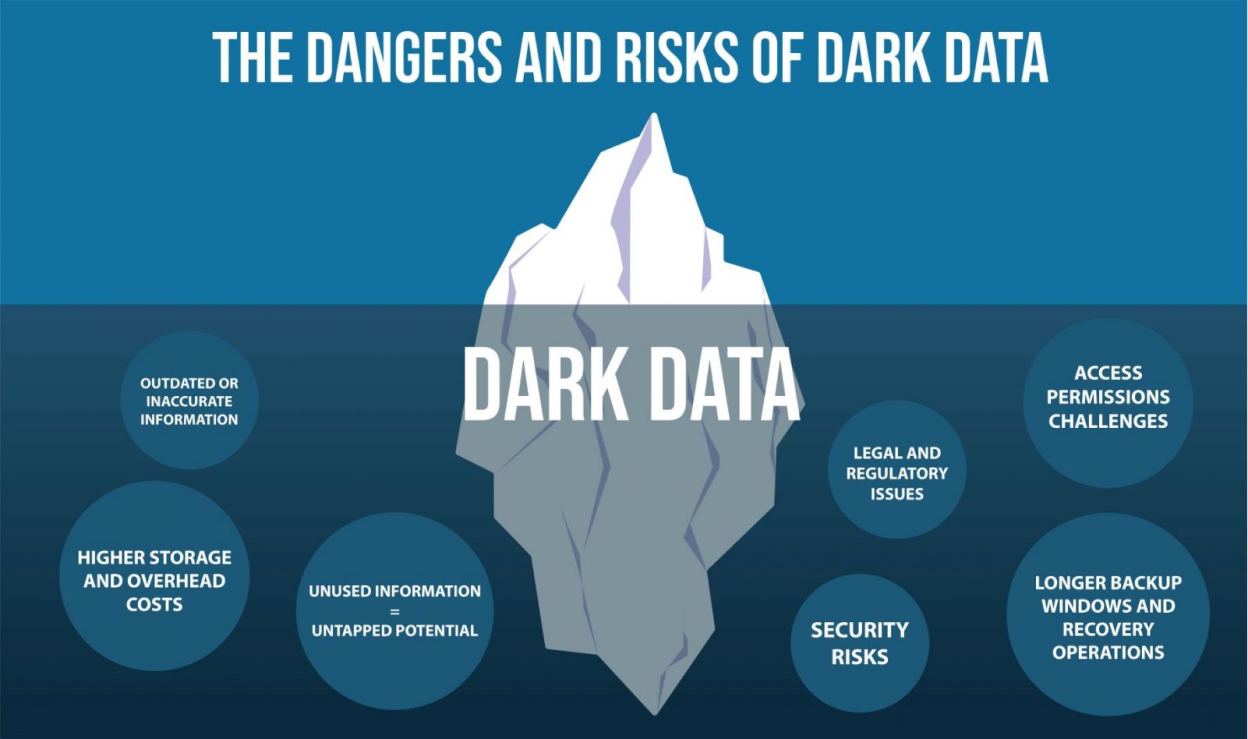
The landscape of Social Media
What is the most significant effect of social media on our lives? The most significant consequence is that we are all constantly linked. If you're wondering how it determines the future of Data Science, consider the massive data that 3.5 billion active users (one-third of the world's population) generate through Facebook, YouTube, Twitter, Instagram, and other platforms!
On numerous social media sites, we express our feelings and even our deepest thoughts. It provides unparalleled amounts of knowledge to the user, which can have a direct impact on a company's strategy.
As a consequence, anytime social media features are changed or upgraded, it results in promotional campaigns & new marketing concepts from firms. Companies must embrace a new analytics approach in this age of dynamics, where data will no longer be static but will be in high demand again for the user insights it delivers.
Without a doubt, implementing a fresh analytics approach will alter the Data Science market trends!
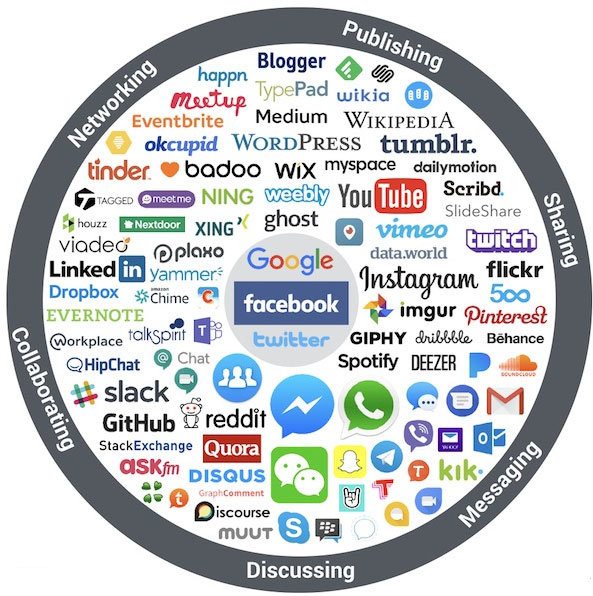
Predictive Modeling
Big data mining and its analytics have constantly been a significant approach for businesses to leverage data and gain a competitive advantage.
But what if businesses could see into the future & anticipate their consumers' next move based on past behavior? This will undoubtedly have an impact on the company's bottom line & outcomes. And predictive analytics enables it.
When it comes to Data Science & Predictive Analysis use scenarios, the best are trip-planning systems that allow consumers to choose destinations, dates, MPM (miles program memberships), and other criteria that determine travel details. These solutions, which include tools that allow consumers to develop their customized models to get the required outcomes, represent a shift towards more user-friendly computer science.
We've also seen significant organizations like Airbnb use Data Science & Predictive Analysis in this way. Airbnb's case study is one of the few Data Science Case Studies that are gaining interest on Google.
The display of these parts of the evaluation process in an incredibly simple, consumer-facing UI, on the other hand, is exactly what destiny holds for data science.
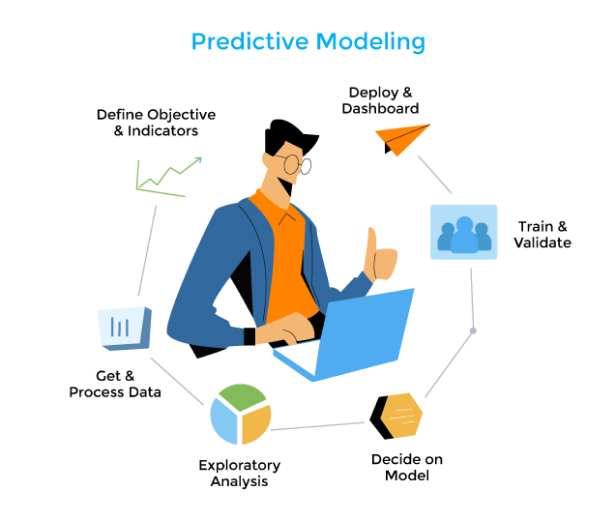
Data Ops
DataOps, a notion that emerged in 2017, is gradually becoming a key discipline for every business to thrive in this growing digital environment. There are three major explanations for this increase:
-
Data engineering pipeline tasks have gotten increasingly complicated, necessitating the use of additional integration & regulation technologies.
-
Generating data cohesion which is a huge effort since many LOBs make intelligent data judgments.
-
Creating an information system is a tedious and time-consuming operation, even in the Cloud.
Data ops use Agile approaches to deliver improved data quality by incorporating all operations from collecting to analytics to forecasting, testing automation, & delivery .
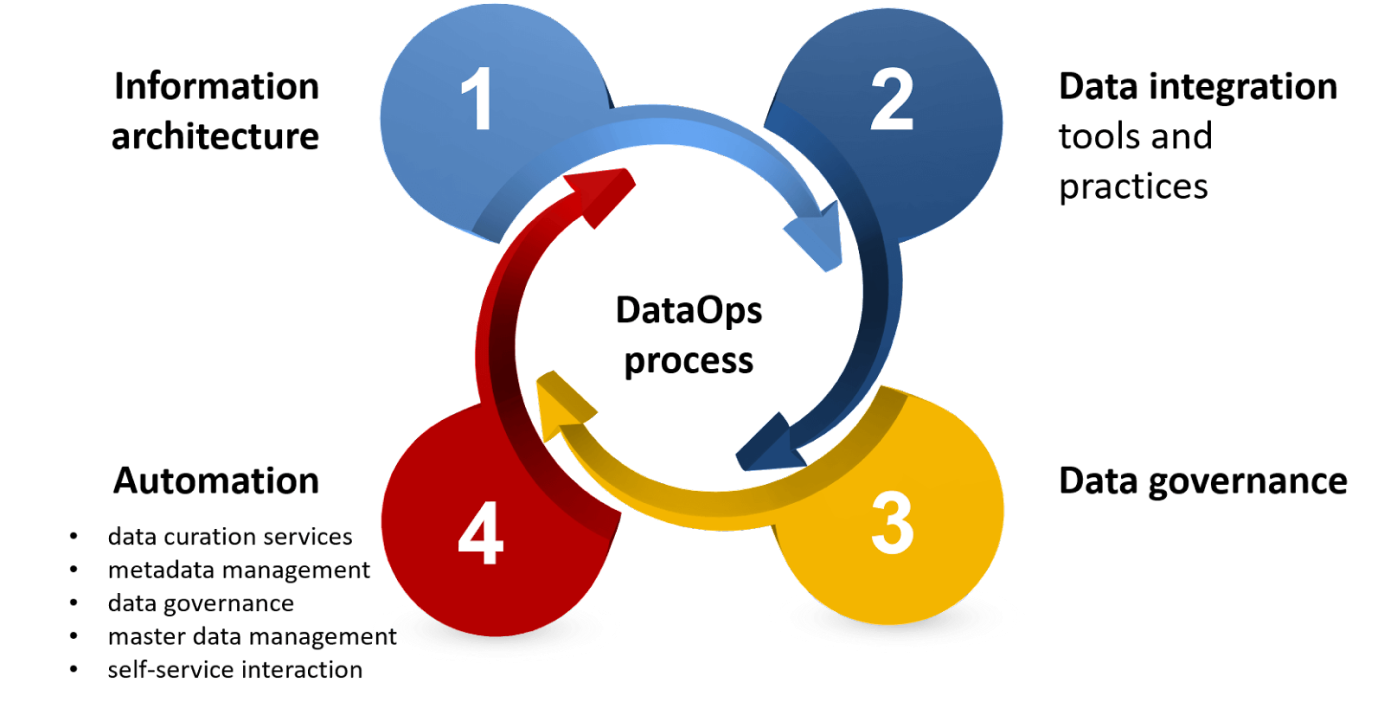
Increase Transparency by Enhancing Insights
As more businesses incorporate data science into their operations, relying on AI-derived advice that limits access to information is now becoming troublesome. The situation has been compounded by increased governmental supervision, such as the GDPR. Transparency observations make AI models more "oversight" friendlier &, as a result, significantly more actionable.
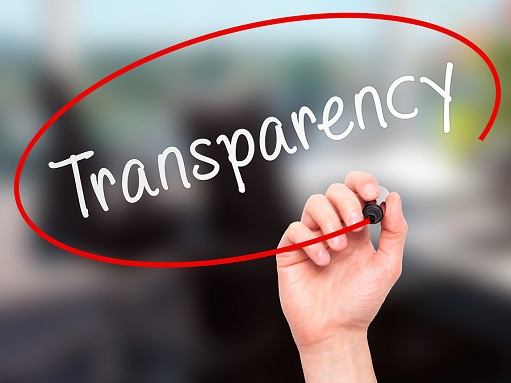
Data Scientists' Skill Sets Are Evolving
It appears that the moment has come to become the latest generation data scientist. Because of improved UX for machine learning, fewer technical skills are essential for many data science roles; however, people in these areas are increasingly required to work throughout silos and become more incorporated into their biz; this can imply that interpersonal skills, elevated concentrations of interaction, and collaborative effort now are essential for a current victory.
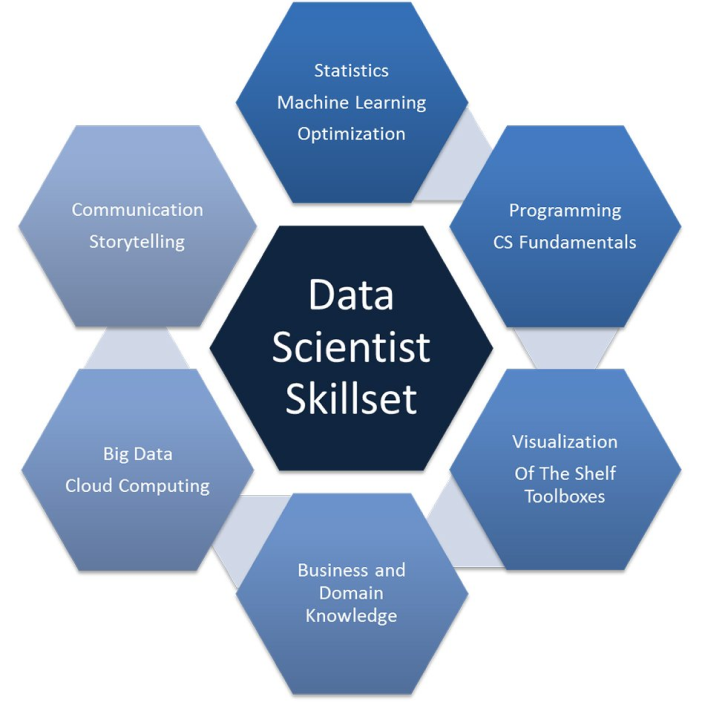
The Influence On Machine Learning Capabilities
5G has several advantages, including increased speed, capacity, and connection. This will give even more data for organizations to feed their machine learning, as well as quicker data transfer from sensors, enabling IoT capabilities. As machine learning takes up the slack, office occupations will become less repetitive.
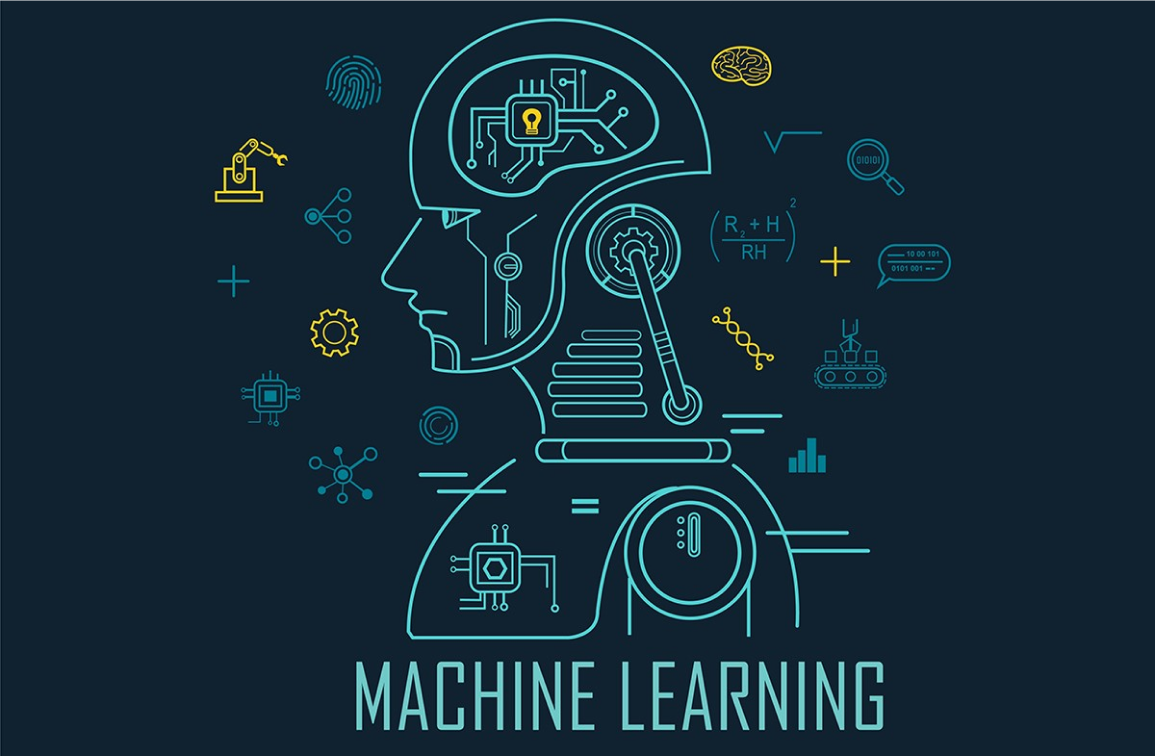
Making Data Useful For Data Science
One of the most significant barriers to data science success is improperly prepared & managed data. CIOs & CDOs should concentrate on enhancing the quality of the data & giving data to data scientists personnel that is appropriate to the current projects & actionable throughout to speed up data science initiatives and decrease failures.
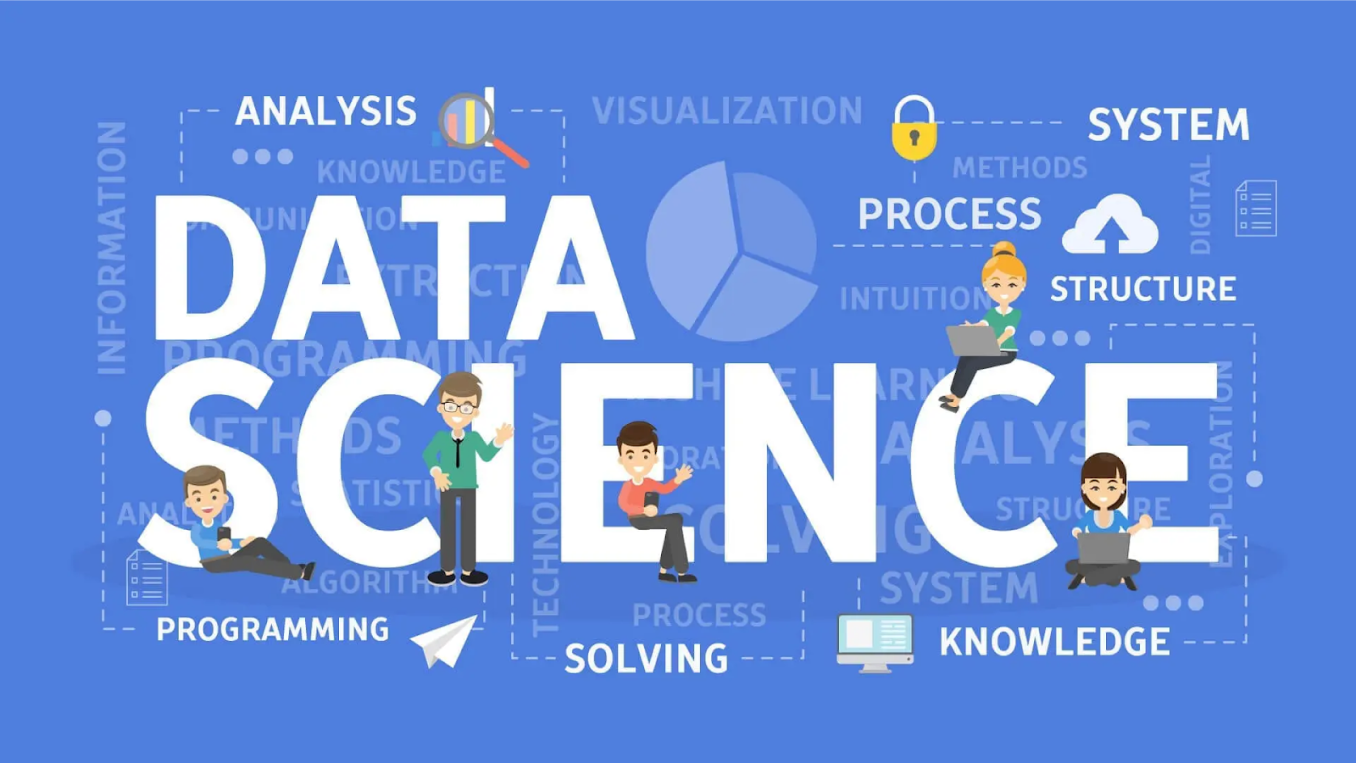
Increasing "Time To Value"
Iterative processes are used in data science. It entails developing "research hypotheses" and thereafter validating them. This back-and-forth strategy incorporates a wide range of professionals, from data scientists to topic matter specialists & data analysts. Organizations must develop ways to speed up the data science procedure to make the "try, test, repeat" cycle quicker and much more reliable.
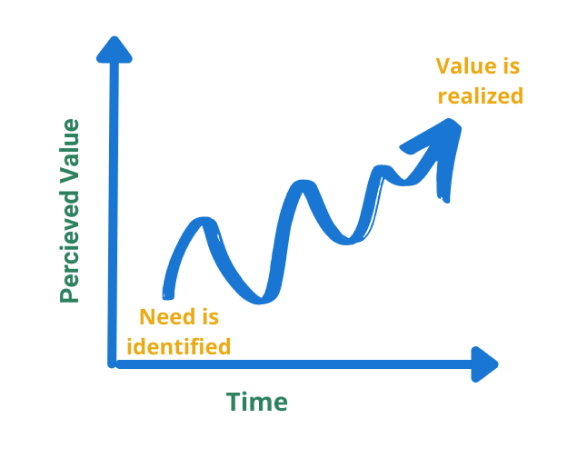
Implement Data Science in Organizations
Despite machine learning models being frequently short bits of code, when they are prepared for production, implementing them can be difficult and troublesome. For instance, because data analysts are not computer programmers, the integrity of their code may be inadequate for operation. Data scientists frequently verify models in lab contexts using downsampled datasets, and algorithms may not be scaled enough for the power generation of datasets.
Furthermore, the effectiveness of deployed models degrades as data changes, rendering model management critical for constantly extracting value creation from AI and ML models. Information & features streams are significantly larger & more complicated than Machine learning itself, but operationalizing them is even more difficult.
One possible method is to use APIs to leverage notions from continuous deployment. Data science automation may produce APIs to perform the whole data science workflow, speeding deployment while also connecting to development platforms to improve model optimization & maintenance.
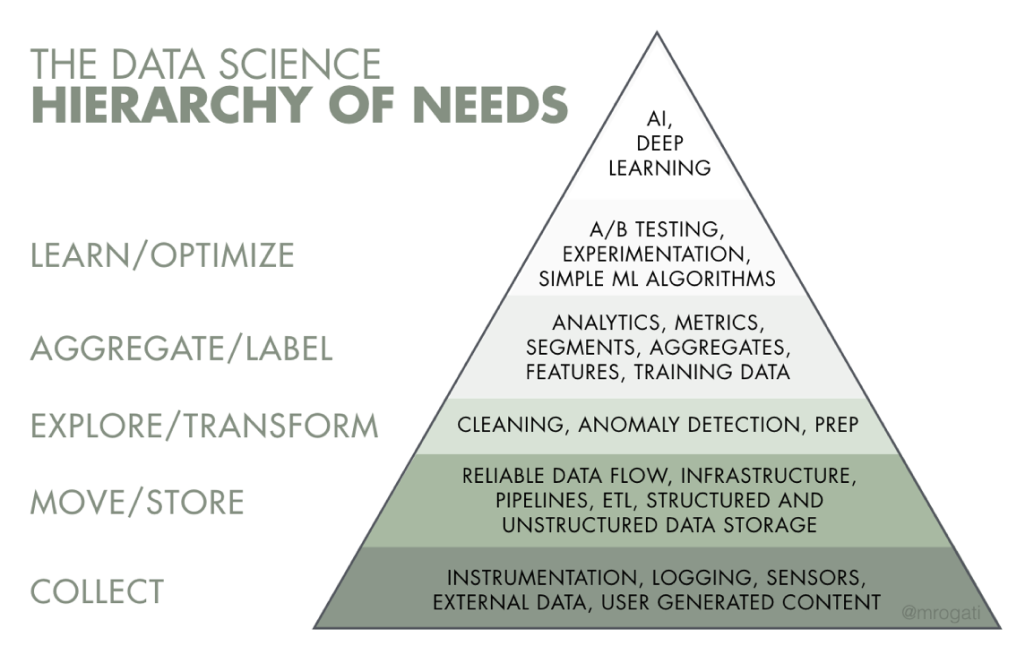
Scalable AI for Company’s Expansion and Speed
Today's enterprises are a synthesis of statistics, system design, machine learning deployments, data mining, & plenty more. For clarity, these elements must be merged into scalable & flexible algorithms capable of analyzing huge amounts of information at an internet scale. Here are some of the reasons why you should learn further about Scalable AI.
-
The capacity of algorithms, statistical models, or infrastructures to work at the scale, speed, & intricacy necessary for the task is defined as scalable AI. Scalability starts to add up to overcoming shortage & acquisition challenges of quality data and can be utilized to enhance data sustainably by recycling & recombining abilities to scale beyond business problem definition while discussing models and administration.
-
To sustain sophisticated ML and AI skills while taking advantage of fast innovation in AI technologies, you will need to build up production & distribution of data pipelines, construct extendable architectures, and current acquisition techniques.
-
Modular algorithms and infrastructure swivel to deploy AI power to vital missions, including centralized data center abilities & decentralized cloud-enabled & network-enabled peripheral device applications.
As easy as it may appear, scalable AI is complex; aligning many scalability elements with the company's conduct of business, assuring increased technical efficiency, lack of integration with data quantities and systems, and, at least, data protection! In 2022, businesses will require professionals to handle these problems and create a unified modeling workflow.
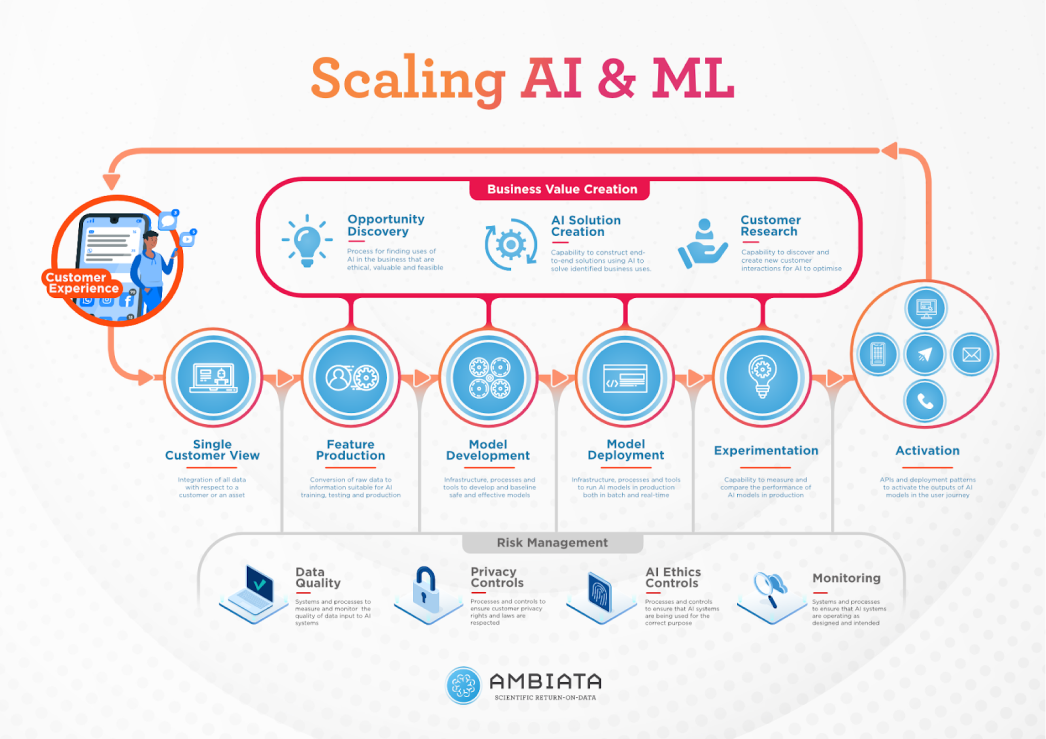
Build An Engaging App For Free
Conclusion
Data science is the heart of AI & machine learning. While the potential of AI is genuine, so are the issues associated with data science. Data scientists can now begin to concentrate on what to resolve, instead of how to solve, thanks to greater planning, tighter collaboration with the lines of business, as well automation of the most laborious & repetitious elements of the process.