In the last two decades, the rapid expansion of technology & AI has radically affected the environment we exist in & the way we engage with it. Researchers have figured out how to save information on how people engage with technology, and take it straight ahead to how the user hovers their mouse. We, humans, are always attempting to guess what other people could do. However, we frequently managed to do this by noticing recurrent hidden tendencies and patterns in their behavior.
When we go deeper into the Internet of Things (IoT), we see intense interaction & mixed connectivity among various devices across the internet in general. As a result, these devices create vast amounts of Big Data.
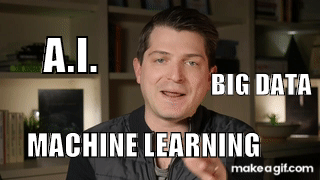
We nowadays are firmly in the big data age, with computers and algorithms gathering and analyzing the specifics of just about everything we do with all those networked gadgets instantaneously or on a real-time basis. Companies consider it as the pinnacle for ultimately predicting who, what, when, and where customers buy their product or services and other offerings, as well as what potential future alternatives can be appealing to them.
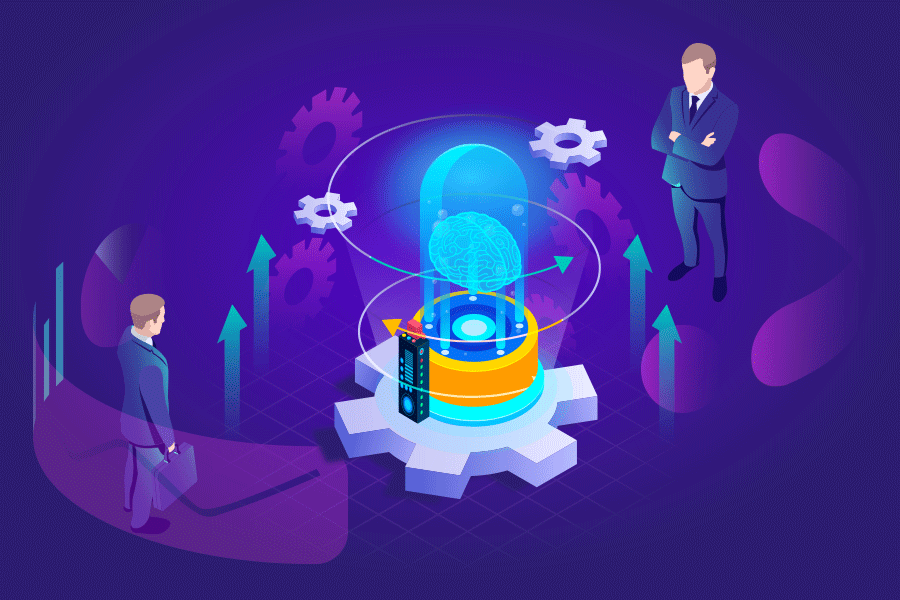
What Is Data Analysis
The concept of data analytics covers the vast field reach by defining it as the act of analyzing raw data to detect trends and answer questions. However, it comprises a wide range of procedures with a variety of objectives. Some elements of intelligent data can aid a range of endeavors. By integrating these elements, an effective data analytics program will present a comprehensive image of what people/audience/customers are doing, where they would have been, and where they can go.
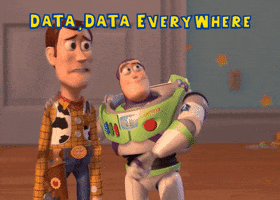
Data analytics generally starts with big descriptive data and statistical models. This is the procedure for analyzing historic patterns and trends over time. This can also be called quantitative data analytics. This historic and quantitative approach seeks to provide a response to the query "what scenarios happened?" This frequently entails calculating traditional measures (ROI). Each industry will have its range of metrics. Descriptive analytics doesn't make forecasts or provide real-time practical suggestions. It is concerned with summarizing facts in a comprehensible and detailed manner.
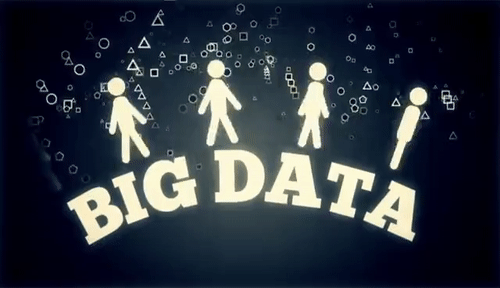
Advanced analytics is made possible by machine learning technologies such as neural network models, natural language, sentiment identification, and others. This information delivers fresh data insights. Advanced ML analytics deals with "what if?" possibilities. The accessibility of neural network models, large data sets, and computer power have allowed these approaches to be used in a variety of businesses. The acquisition of large data sets is critical for implementing these strategies. Big data analytics enables organizations to derive relevant insights from vast and extensive and diverse data sources.
Build An Engaging App For Free
What Is Behaviour Data and Behaviour Analysis
Behavioral data, also called cognitive data is generated by or in reaction to a customer's interaction with an organization. Page visits, newsletter sign-ups, as well as other important user behaviors, are examples of this. Websites, smartphone applications, CRM systems, and marketing systems are all common sources of behavioral data.
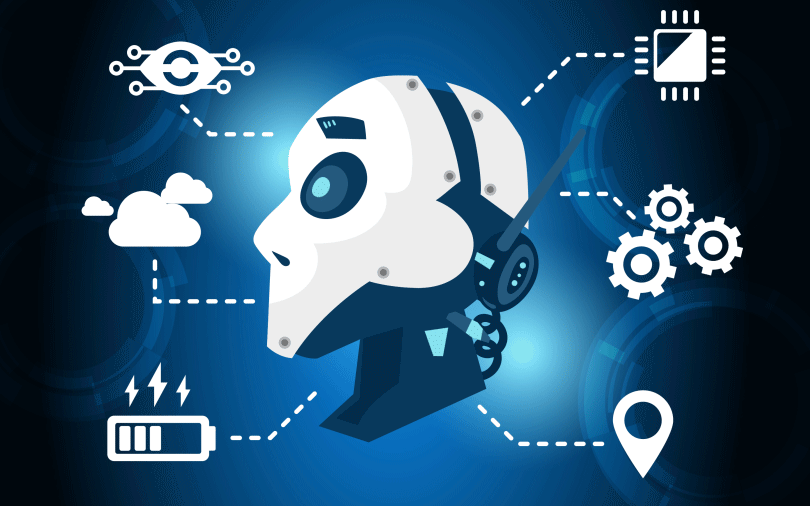
Customers might be persons, corporations, or consumers, but behavioral data can always be traced back to a specific end-user. The process of doing this is known as behavior analysis. It should be noted that this user might be either a recognized person (logged in) or anonymous (not logged in).
For Instance- It is critical to grasp how AI may play a significant role in estimating and comprehending how people behave during any specific event. It is behavioral data that can be acquired without statistical sampling. Notable examples of smartwatches working as sensing devices not only provide the necessary information but also reduce the time that otherwise would have been spent obtaining that type of data. It's like an intuitive input that is unrelated to testing, collecting manual data, and then making out paperwork. Many efforts to monitor stress or wellness are already in existence.
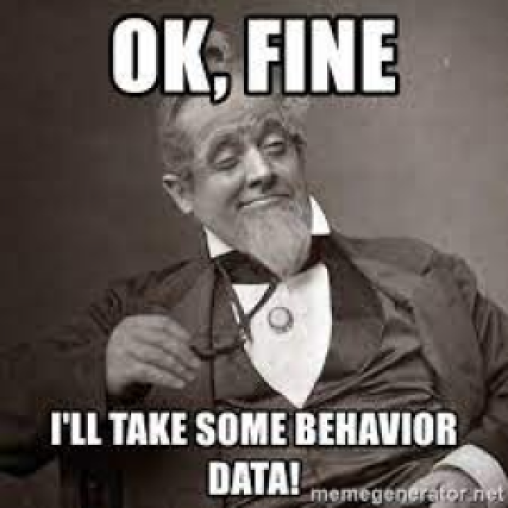
What Can Data Tell Us About Human Behaviour
Companies are investigating the use of IoT in conjunction with behavioral analytics to supplement their current productivity rate. Businesses are seeking IoT, ML, AI to better their workflows because of the possibilities of lower costs, more precise delivery estimates, and greater product quality and development, seeking business opportunities and a lot more.
Datafy customers/visitors sentiments & emotions- The rise of constant self-reporting on the internet has generated lots of consumer personal data. Many marketing intelligence firms now leverage this data by scraping the web for detailed illustrations of public attitudes towards certain problems, brands, goods, and services.
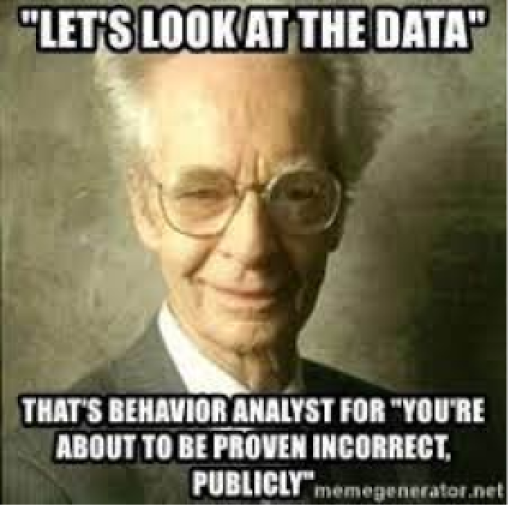
Datafy Interactions- We can now monitor and evaluate not just how individuals connect, but also how, when, and with whom they relate. By datafying work and social relations on a worldwide scale, the social media scraping method has the potential to alter our perspective of relations and interactions.
Datafy Speech- Speech analytics is growing more popular, especially since chats are increasingly being recorded and saved as part of engagements with contact centers and with one another. As deep learning advances, the amount of audio data and significance that can be collected in an understandable way expand.
Datafy Culture- Cultural analytics is a brand-new field that analyzes the picture and video collections using image processing and visualization to uncover cultural patterns.
Datafy Personalization: Some businesses employ marketing technology to maximize the predictive aspects of their data. Marketing departments, in particular, require these sorts of insights due to sales process challenges caused by the multichannel consumer experiences generated from online and mobile app environments.
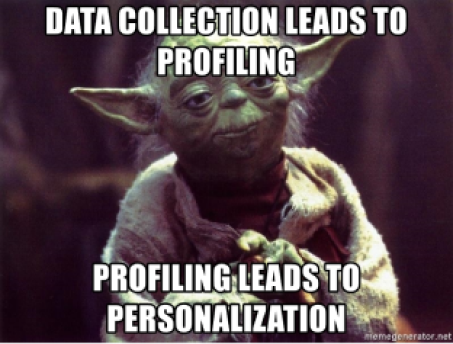
Datafy Conversion Rate- When it comes to CRO, it's important to eliminate preconceptions and guesses and make all decisions based only on customer behavioral data. Collect and monitor your mobile app data on a regular basis to understand more about your app users and their interests. This data should help you decide where to focus your optimization efforts.
Datafy Segmentation- Customer segmentation is the practice of classifying clients based on similar characteristics in marketing. Understanding client buying behaviors allows you to sell more effectively. For example, it indicates the sizes of the various industries, how much money we generate from them, and so on. This can aid in determining how to allocate the money on marketing.
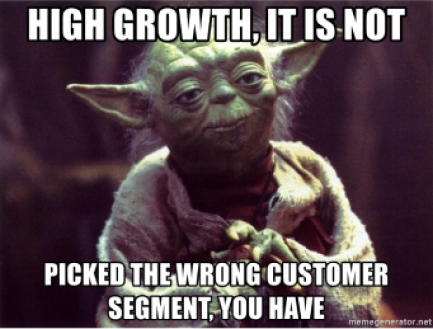
Datafy Product Recommendations- This is another kind of behavioral data that can be known as client order data, and in which we are particularly interested in purchases of the customers in connection with some other items and then as per the purchase intend to send customers relevant product recommendations.
ML Block- We can learn about users' purchasing intentions by using behavior analytics. The data also assists us in uncovering hidden interests. We may generate segments using page visit statistics. Segments assist us in understanding buying intent. We may send reminders, suggestions, and offers and incentives to the consumer based on purchase intent, which can result in greater order values and recurrent orders.
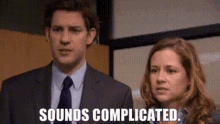
You think is this too complex to program? No issue, swipecart's ML blocks perform everything we discussed above. Well, these are just a few, yet there are many more.
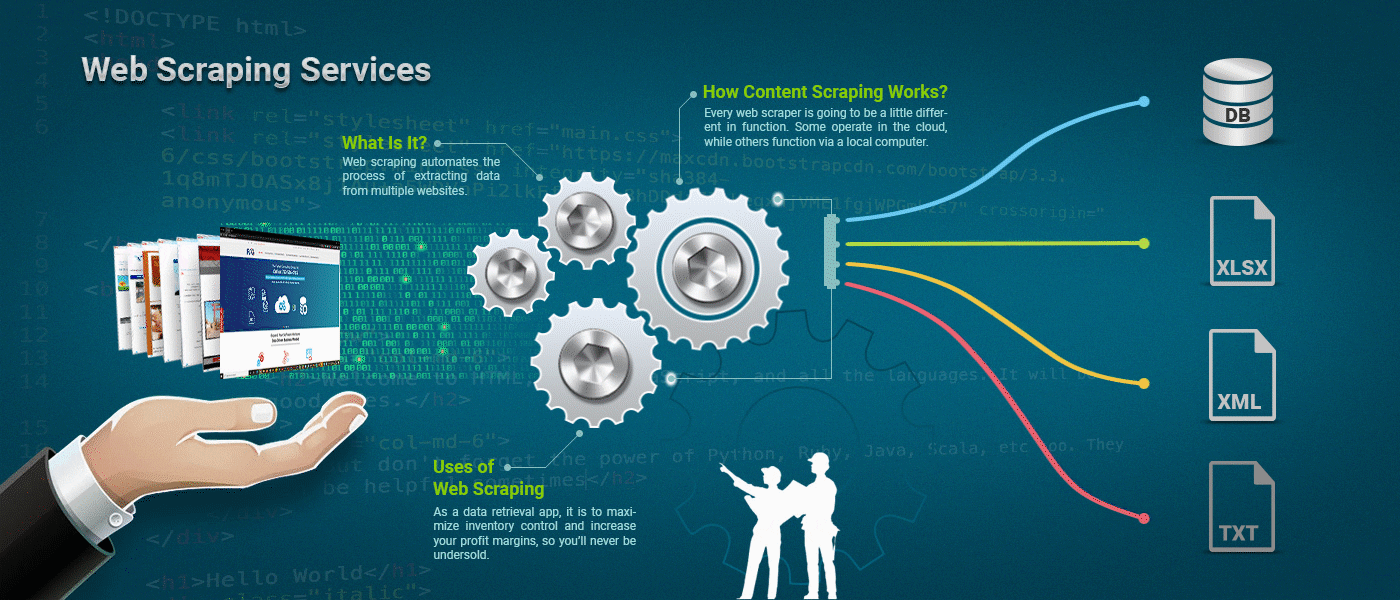
Big Data and Behavioral Analytics
When data analysts or statisticians implement procedures that need a significant quantity of data, this is referred to as big data. In principle, greater data enhances the usefulness of statistics, given the data quality remains constant. Many of the most sophisticated algorithms, such as neural network models, are unsuccessful with little quantities of data but can turn significantly more efficiently with massive volumes of data.
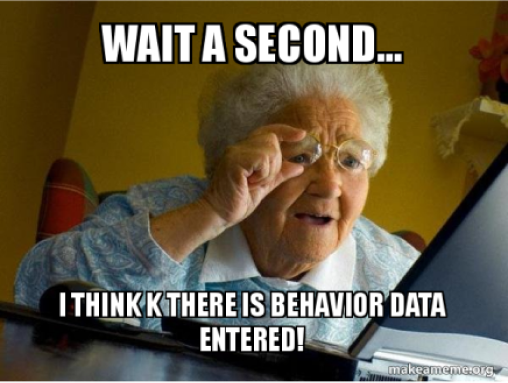
Artificial intelligence has already established the standard in just about every other discipline and now is ready for behavioral science. A human's mental process influences other individuals' behaviors, which accumulates lots of data for later study. Psychology may be found everywhere, not alone in clinical terminology, but mainly in our daily lives. What a buyer purchases at a given store also obviously influences human behavior. Everything is tied to behavioral science. You can now know your customers' purchasing habits and response patterns to sleeping patterns and a lot more. However, thanks to big data, this branch of study has only become stronger.
As we're more data-connected both online as well as offline, there is no doubt that our digital waste will reveal more and more about us, enabling improved short-term forecasts of our purchasing patterns and interests. However, forecasting future demands and behavior is a considerably more difficult task. As a result, I believe that humans will continue to drive big data in organizations instead of the other side for a little while.